Unleashing the Power of AI Agents: A Comprehensive Guide
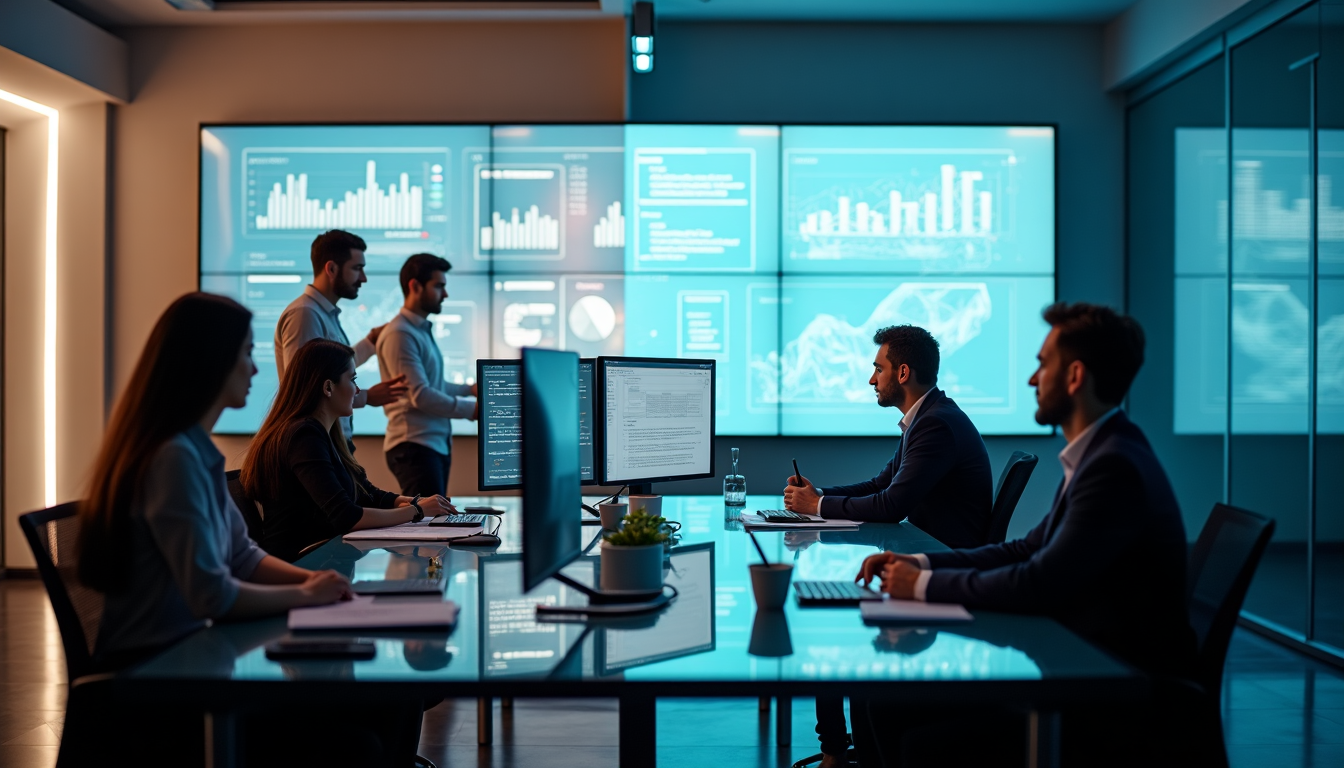
AI agents represent a $3.86 billion market that's projected to grow at an astounding rate of 45.1% annually from 2024 to 2030. This remarkable growth shows a major shift from simple chatbots to sophisticated systems that automate complex workflows, monitor data streams, and execute tasks without constant human supervision.
AI agents are changing businesses of all types. Healthcare organizations make their revenue cycle tasks more efficient, while software development teams use them to diagnose system performance issues automatically. Microsoft 365 Copilot already serves as a personal assistant and handles everything from daily duties to creative projects.
These AI agents revolutionize business operations by knowing how to work continuously. They handle tasks from reviewing customer returns to managing shipping invoices. Recent breakthroughs in memory and tools enhance their autonomy. They maintain context across multiple actions and deliver better results in different applications.
Evolution of AI Agents Technology
AI agents have transformed dramatically since their early days in artificial intelligence. The original systems were simple rule-based programs that performed specific tasks without learning capabilities.
From Simple to Complex AI Agents
The experience started with simple reflex agents that responded to environmental stimuli using predefined rules. Model-based agents came next and introduced internal representations of their environment. Goal-based agents followed and could assess different scenarios to achieve specific objectives. On top of that, utility-based agents brought sophisticated decision-making capabilities by measuring how desirable different states were.
Key Milestones in AI Agent Development
Several breakthrough moments altered the map of AI agents. Deep Blue made history in the 1990s as the first program to defeat a world chess champion. Siri's launch in 2011 brought AI agents into everyday life. The rise of large language models and advanced memory management systems has enabled AI agents to maintain context through multiple interactions.
Current State of AI Agent Technology
The AI agents market valued at $3.86 billion shows promise with 45.1% annual growth expected from 2024 to 2030. A Capgemini survey reveals that 10% of firms now employ AI agents, and 82% plan to add them within three years. Modern agents show remarkable capabilities:
- Autonomous Decision-Making: Agents process inputs, make decisions, and execute actions independently
- Memory Management: They track conversations short-term and store information long-term through vector databases
- Tool Integration: They know how to access and use various digital tools and APIs to complete tasks
Today's AI agents excel at complex tasks in healthcare diagnostics and financial analysis. The technology has moved beyond simple chat-based interactions to action-based systems that execute sophisticated processes. These advances have made AI agents essential parts of modern business operations, showing remarkable progress from their modest beginnings.
Strategic Planning for AI Agent Implementation
AI agents work best when organizations plan well and line up their approach with business goals. Note that organizations need a full picture of their operational setup before they start using these technologies.
Assessing Business Needs and Requirements
Support profiles and query volumes are the foundations of successful AI agent deployment. Teams should review their daily query volumes and support channels to figure out how much automation they need. A content audit of existing knowledge helps create a strong base for AI agent training.
Choosing the Right AI Solution
Finding the right AI solution means looking at several factors that match organizational goals. The system's ability to scale lets the AI agent handle bigger datasets and workloads effectively. Data encryption and access controls are vital parts of security that need attention.
Key evaluation factors include:
- Integration capabilities with existing infrastructure and workflows
- Customization options for specific business requirements
- Economical solutions that balance upfront costs and running expenses
- Performance reliability and consistent results
Building the Implementation Roadmap
Teams use a structured "Crawl-Walk-Run" approach to implement the system. Most organizations start small with 1-3 manageable use cases that show clear value. This method helps teams gain confidence as they reshape the scene with AI.
Quarterly Business Reviews (QBRs) help track ROI and spot areas that need work. Strong monitoring systems track performance metrics and see how well users adopt the technology.
Change management deserves attention in the roadmap since success depends on more than just technology. Organizations need good training programs and support systems to blend with current workflows smoothly. Teams that follow structured processes end up more satisfied with their AI agent systems.
Creating Custom AI Agents
Custom AI agents need careful architectural design and thorough testing protocols. Organizations prefer building custom AI agents over pre-built solutions to meet their specific business needs.
Designing Agent Architecture
Two core components make up an AI agent's foundation: an LLM planner for action sequencing and specialized tools that integrate external resources. These components enable autonomous decision-making and task execution. A well-laid-out architecture should have:
- Systems that manage memory and retain context
- Tools that access external resources
- Mechanisms for making decisions
- Modules that break down tasks
- Systems that provide feedback for improvement
Training and Testing Methodologies
Custom AI agents require a structured training approach that combines supervised learning with reinforcement techniques. Simulation environments let teams safely test AI agents by copying ground conditions without risks. Automated testing frameworks help make evaluation quick and adaptable.
The Model-Based Transfer Learning (MBTL) algorithm works five to 50 times faster than standard approaches when training AI agents. This method picks specific tasks that boost overall performance, especially when you have complex scenarios like traffic control systems.
Integration with Existing Systems
Connecting custom AI agents with current infrastructure brings its own set of challenges. Legacy systems often face data compatibility issues with AI agents. Up-to-the-minute data analysis needs reliable infrastructure that handles high-throughput data streams.
Organizations can tackle these challenges with a multi-layer approach that has:
- Protocols that isolate data and protect enterprise information
- Mechanisms that filter content for appropriate responses
- Systems that monitor and refine agent settings continuously
Human oversight plays a vital role to ensure AI systems line up with ethical standards and user expectations. Organizations must maintain complete test coverage as they scale AI agent deployments. This helps catch potential behavior, performance, or security problems early.
Maximizing ROI with AI Agents
A step-by-step method helps measure both tangible and intangible benefits when evaluating how AI agents affect finances. A McKinsey study shows that 42% of AI-adopting companies report reduced costs. The study also reveals 59% of companies achieved successful revenue growth.
Cost-Benefit Analysis
The money effects of AI agents show up at different times. Companies usually see quick cost reductions through task automation in the first 6 months. Customer satisfaction and operational efficiency improve during months 6-20. AI agents prove their strategic value after 20 months by creating new business models that propel development.
Performance Metrics and KPIs
Companies just need to track multiple indicators to measure how well AI agents work. The core metrics include:
- System Performance: Uptime percentage, response times, and error rates
- Operational Efficiency: Task completion rates, automation levels, and resource utilization
- Business Impact: Customer satisfaction scores, resolution times, and cost per interaction
Agent performance analytics helps companies get the most from their AI investments. Companies can spot areas to improve and refine their AI systems through detailed measurement and evaluation.
Optimization Strategies
Companies must use targeted approaches to improve ROI. We analyzed performance patterns to predict future trends and resource needs. The systems work better with constant monitoring that allows quick adjustments.
Informed performance dashboards affect company KPIs greatly. About 70% of respondents agree that evolving KPIs using AI drives business success. Companies that use well-laid-out processes to monitor AI agent performance report higher satisfaction rates.
Predictive analytics helps businesses spot potential bottlenecks and optimize resources. The original investments can be big, but organizations using AI agents report cost savings of up to 90% compared to traditional methods.
Overcoming AI Agent Implementation Challenges
Organizations must systematically tackle unique challenges when implementing AI agents. Studies show up to 70% of change initiatives fail because of various implementation obstacles.
Common Technical Hurdles
Resource management is the biggest problem in AI agent deployment. Organizations don't deal very well with computational demands, especially in tasks with machine learning and data processing. Response times and overall performance suffer as user numbers increase, which makes latency issues more evident.
AI agents just need vast amounts of high-quality data to train and make inferences. Notwithstanding that, many organizations face these challenges:
- Data silos and fragmented information
- Inconsistent data standards
- Quality issues including missing values
- Privacy and security concerns
Change Management Considerations
AI agent implementation works best with an all-encompassing approach to change management. Employee concerns about job security and disruption to optimized workflows often create resistance. Organizations should guide their teams through this transformation experience.
Early-adopter outreach programs build acceptance effectively. Teams should engage non-adopters early in the implementation process to identify potential biases and resistance points before they grow. Centers of Excellence (CoE) become vital after implementation to centralize expertise and codify best practices.
Risk Mitigation Strategies
Robust security measures protect AI systems from various threats right from the start. Organizations must prioritize data privacy, model integrity, and compliance with regulations like GDPR and HIPAA. This work includes:
Role-based access control mechanisms ensure proper data handling and system access. Network segmentation and agent isolation contain potential breaches in previously siloed systems. Organizations must implement strict isolation protocols and regular session state cleanup procedures, though it's challenging.
Strategic measurement is vital to risk mitigation. Usage rates, retention, and user feedback give an explanation about system utilization and friction points. Real-time monitoring systems detect anomalies and potential security breaches in AI deployments.
Whatever the implementation approach, organizations must set clear guardrails. These guardrails act as safety protocols that prevent AI from causing harm, making biased decisions, or being misused. They help maintain consistent tone, quality, and context across all AI-generated responses and deepen their commitment to brand identity.
Conclusion
AI agents are pioneering technological advancement and reshape how businesses operate. These systems have grown from simple rule-based programs into autonomous entities that mark a crucial milestone in AI development.
Companies achieve remarkable results when they plan their AI agent strategy with care. The numbers tell the story - successful implementations cut costs by 42% and boost revenue by 59%. These results show how properly executed AI solutions make a real difference.
Technical hurdles exist, but organizations overcome them through methodical work. Success comes from managing resources well, handling data properly, and implementing strong security. Teams adapt to new ways of working through effective change management that keeps efficiency high.
AI agents paint a bright future ahead. They handle complex tasks on their own and keep track of context between interactions, which makes them vital for modern businesses. The path to success needs careful planning, systematic implementation, and constant fine-tuning of these powerful tools.